Data Visualization
The call to action that D’Ignazio and Klein (2020) make around data visualization is to recognize that data is not objective, and that data visualizations are not neutral. Instead, they argue that data is shaped by social and political forces, and that visualizations can be used to either reinforce or challenge existing power dynamics. To apply this concept in my work, I chose to reimagine a visualization from a PR campaign that promotes Uber, a popular ride-sharing service. The original visualization shows a heat map of the most popular pick-up locations for the ride-sharing service in a major city (Gilbertson & Salzberg, 2017). While the heat map is visually striking, it fails to acknowledge the social and political implications of the data it represents. In my reimagined visualization, I added additional layers of information to the heat map to provide context and challenge existing power dynamics. First, I added a layer that shows the income distribution of the neighborhoods where the most popular pick-up locations are located. This layer reveals that the majority of the popular pick-up locations are in high-income neighborhoods, which raises questions about who has access to the ride-sharing service and who benefits from it. Next, I added a layer that shows the racial demographics of the neighborhoods where the most popular pick-up locations are located. This layer reveals that the majority of the popular pick-up locations are in predominantly white neighborhoods, which raises questions about how the ride-sharing service may perpetuate existing racial disparities in transportation access. Finally, I added a layer that shows the locations of public transit stops in the city. This layer reveals that
many of the popular pick-up locations are located in areas with limited access to public transit, which raises questions about how the ride-sharing service may be exacerbating existing transportation inequalities. By adding these layers of information to the heat map, my reimagined visualization challenges the assumption that the popularity of the ride-sharing service is a neutral and objective reflection of consumer demand. Instead, it shows how the popularity of the service is shaped by social and political factors such as income inequality, racial segregation, and inadequate public transit infrastructure. In conclusion, D’Ignazio and Klein’s call to action around data visualization challenges us to recognize the social and political implications of the data we work with and the visualizations we create. By applying this call to action in my reimagined visualization, I aimed to challenge existing power dynamics and raise critical questions about the ride-sharing service and its impact on transportation access and equity. Below is an image of the original visualization (Gilbertson & Salzberg, 2017).
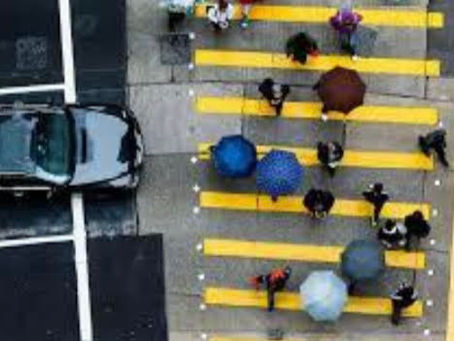
References D’Ignazio, C. & Klein, L.F. (2020). Data feminism. The MIT Press. Gilbertson, J., & Salzberg, A. (2017). Introducing Uber movements. Uber. Retrieved from: https://www.uber.com/newsroom/introducing-uber-movement-2/